I have been studying the SaaS model in depth for almost 7 years now. Since the day Harry Weller walked into my office with a stack of materials and told me to study it and then SaaS build models and bring them to portfolio companies, I don't think a day has gone by where I have not thought about the conceptual and operational nuances of the recurring revenue business model.
Somewhere around mid 2015 I had my "aha" moment in my research when I tied my academic training in mechanical engineering to the startup business models I was building: it's all calculus. The integral-derivative relationship applies incredibly well to the ARR and Recognized Revenue relationship. Making this connection between engineering math and financial math gave me a feeling that only a true nerd could appreciate: the joy of putting integral symbols and accounting terms on the same slide.
The simplest way to illustrate this mathematical parallel is with a car:
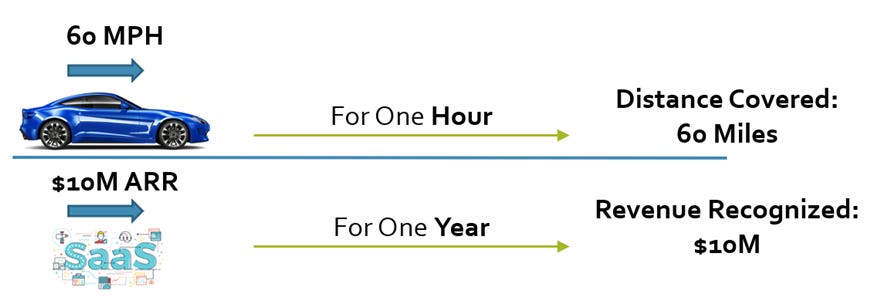
If a car is moving at 60mph, then in one hour it will travel 60 miles (assuming its speed does not change). That is the definition of "miles per hour." ARR is very similar: if a company is "moving" at $10M ARR, then in one year it will recognize $10M of revenue (assuming everything stays consistent). Recognized revenue is the distance, ARR is the speed. It's critical to recognize that ARR is a rate at a specific point in time used to imply something (here, expected recognized revenue in the future period).
For the more accounting oriented, another analogy can be made to the balance sheet:
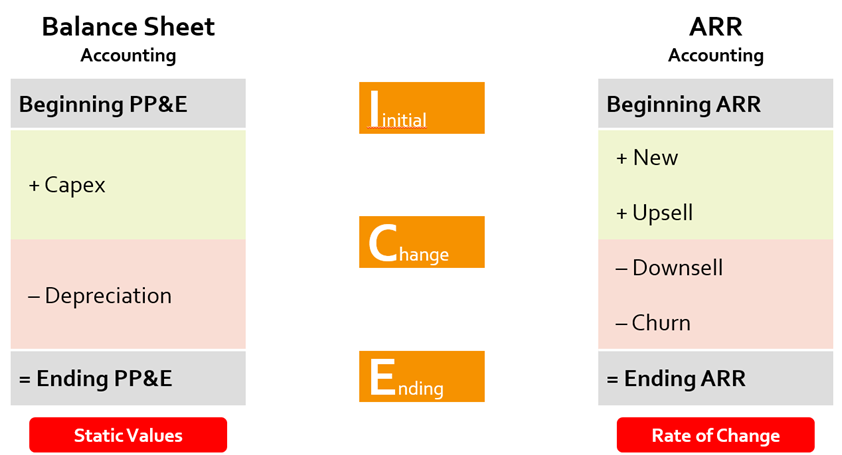
While revenue is the top line metric on the income statement, ARR works more like a balance sheet metric: it is taken at a single point in time rather than over a period of time. This can make income statements confusing and misaligned - another example of the divergence of accounting in economics in subscription businesses.
Now back to calculus... if the ARR function was actually a mathematical equation, you could integrate it. If y = 10x where y = MRR and x = time in months, then after two months MRR = $20. After 12 months, MRR = $120 (assuming we start from $0 of MRR). So at the end of a year, the business has grown from $0 to $120 in MRR. But what is the recognized revenue? The complex answer is that it's the integral of 10x from 0 to 12 months. (Apologies in advance if this triggers a high school calculus flashback.)
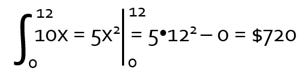
You could also chart this out and see that it is a right triangle with the area of 1/2*base*height. Where the base is 12 months and the height is $120: $1,440/2 = $720).
Pretty cool relationship and calculation conceptually, but in real businesses ARR growth doesn't fit a simple equation (or any equation at all), so it's not inherently practical to start breaking out the power rule and your old textbooks to predict ARR growth.
If we switch back to the car analogy, it takes on a little more of a nuanced meaning. Just like a business doesn't grow on a smooth curve, car speeds do not either. Just like the gas pedal makes the car go faster and the brake pedal & friction make it go slower, so too in a SaaS company, the new sales are making the speed/ARR increase and the churned customers are making the speed/ARR decrease. I will dive into more details in later posts, but the goal in a car is to go as far and fast as you can while burning the least amount of fuel. So too in a SaaS company, the goal is to have the highest ARR you can, recognize the most revenue and burn the least cash. You can think about SaaS Magic Number like the fuel efficiency of a SaaS business - looking forward to diving into why this is actually helpful in building a company.
Please reach out with any questions or comments.
Great piece! Another topic of consideration for further demonstration of this concept are Riemann sums and discrete integrals. Those are what you'd want to use to model the relationship between ARR and revenue because they involve iterative step calculations (MRR and ARR are calculated in finite intervals of 1 mo and 1 yr) as opposed to integrals on continuous functions. But I also think continuous functions are sufficient for creating useful estimations.
To your point at the end of the article; continuous functions may not be sufficient models for real-life business phenomenons, but they are definitely good proxies. This is esp true for larger data sets (i.e. mature public companies). Do you think this approach is equally useful for young startups doing internal modeling?
This is the level of finance nerdery I aspire to