Modeling SaaS with Causal — Answering key Operating Questions
I recently started working with the team at Causal, which has created a powerful browser-based modeling tool. As an excel junkie for many…
I recently started working with the team at Causal, a powerful browser-based modeling tool. As an excel junkie for many years, I see a few key limitations to excel: live data integration, visual sensitivity analyses, easy to manage inputs/outputs, and most importantly, accessibility and understand-ability for non-experts.
Note: I started this post before the markets were affected by the pandemic and think that these issues went from being a “nice to have” for many companies focused on growth to a “must have” in a time when capital efficiency and understanding the levers in your business model needs to become the top priority to make sure you have the best shot at long term success.
Causal allows you to easily isolate key inputs and outputs of the model and visualize the dependencies within the model. This enables what I call “institutional knowledge transfer,” which is the process of explaining expert work inside an organization in order to understand, replicate and ultimately operationalize that work across the organization.
This model is an operational SaaS model. I built it to help operators understand their businesses — bridging the gap between intuition and calculation. While I include all the VC-oriented metrics and ratios as outputs, the purpose of this model is not to assess the health of a business for investment. This model allows operators to put their hands directly on the levers of their businesses and understand how those inputs effect key output metrics.
It is critical for an operator to identify and understand the levers in their business. As new business models come into the world, gut instincts are no longer sufficient to drive businesses forward efficiently. Building an effective model shouldn’t be easy, but Causal helps you make it simple and allows you to visualize your business, and then share it so that others can understand it and provide input.
Now for the Model:
When building a model, it’s important to start with a list of questions that you want to answer. For example:
How much cash do we need based on our current operating metrics?
How does the sales cycle length effect EBITDA?
If churn doubles, where will our ARR be in 2 years?
If it costs $X to acquire a customer at $Y MRR and takes Z months, how long do we need to keep the customer in order to maintain positive unit economics?
To create a useful model, you need to first identify the input and output variables. The output variables should answer the key questions and the input variables need to represent two areas: the ones you control and the ones that control you. Causal allows you to explain these interdependencies visually.
Some of the coolest features of Causal:
Input ranges. For example “$1,000 to $1,500" or “1% to 2%”
Live integration with Google Sheets and Stripe
Automatically updating dates for historical and projected
Create and save multiple scenarios
Group and customize variables
Sensitivity analyses that show the relative impact of multiple variables on a single output
Visual cues for dependencies
What do you need to make it work? Some fairly detailed stats on your business operation including details on customers, mrr, expenses, sales cycle. You’ll also need a view on how these metrics will change over time, but that’s one of the best things about the model — you’ll be able to see what is realistic and how it impacts your bottom line.
Check out the model here:
Operating SaaS Model - Causal
Causal is a browser-based modelling tool to replace spreadsheets.causalapp.com
Also including a link to the super simple SaaS model that I built in 15 minutes here: https://my.causal.app/models/1764
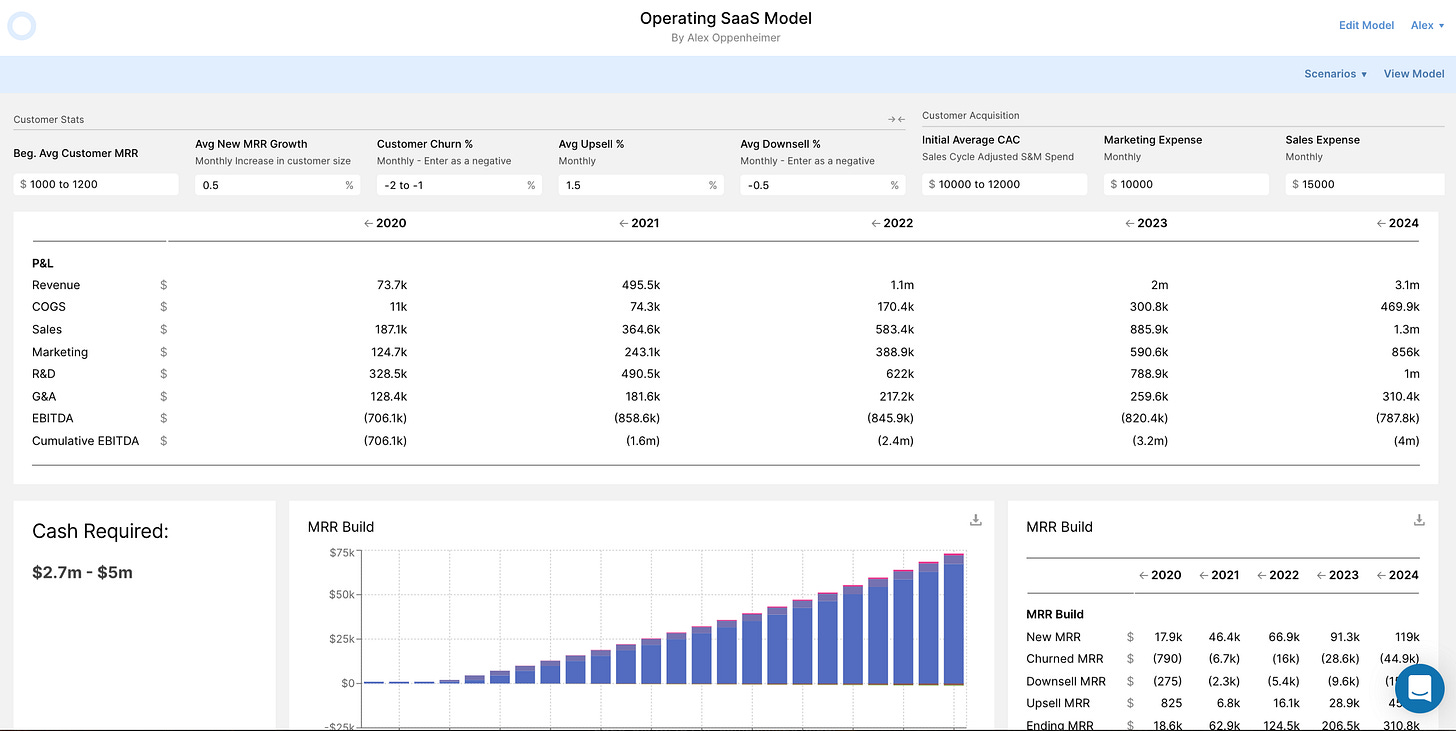
In the name of rigor, I will go through the various definitions I use in this model that will allow you to enter your own company data and instantly gain insight into future operations.
Understanding the inputs…
Customer Stats:
Starting Average New Customer MRR — initial value for new customer ARR
Average New MRR Growth — increase in the size of an average new customer
Customer Churn % — monthly logo churn
Average Upsell % — monthly average upsell/upgrade as a % of beginning MRR
Average Downsell % — monthly average downsell/contraction as a % of beginning MRR
Customer Acquisition:
Initial Average CAC — how much it costs to acquire a customer. This requires an understanding of sales cycle in order to properly attribute S&M spend to each cohort of customers
Initial Marketing Expense — beginning marketing budget
Initial Sales Expense — beginning sales budget
Sales Cycle Length — length of time from initial contact to final sale. For example, if the sales cycle is 3 months and the deal closes in June, then sales expense from June, May and April is averaged and added to the marketing expense from March to get the fully attributed CAC
Average CAC Growth — increase in average customer acquisition cost (i.e. a decrease in sales efficiency) This happens naturally as businesses scale (except when they are viral)
Initial S&M Spend Growth — initial growth rate of the S&M budget
S&M Spend Growth Deceleration — the gradual decrease in the growth rate of the S&M budget
Current Business:
Current MRR — the MRR at the end of last month
Current Number of Customers
Other Expense Metrics:
Gross Profit %
R&D Expense
G&A Expense
R&D Expense Growth % (Monthly)
G&A Expense Growth % (Monthly)
Take a look and feel free to reach out to me and the Causal team with any questions and feedback.
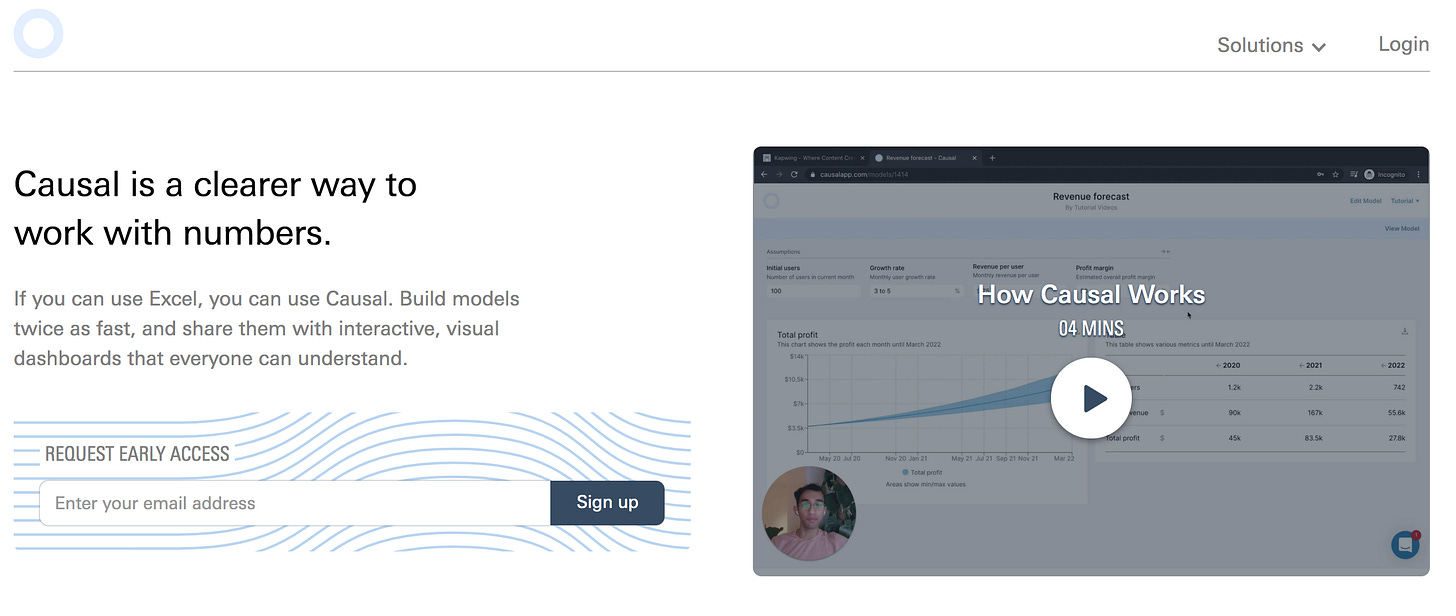